What You Need to Know about Data Mining and Data-Analytic Thinking
ByFoster Provost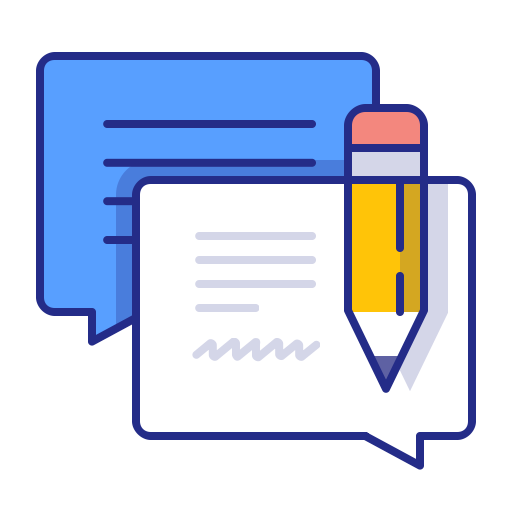
★ ★ ★ ★ ★ | |
★ ★ ★ ★ ☆ | |
★ ★ ★ ☆ ☆ | |
★ ★ ☆ ☆ ☆ | |
★ ☆ ☆ ☆ ☆ |


Check out Audiobooks.com
Readers` Reviews
★ ★ ★ ☆ ☆
janie
although billed, at least in part, as aimed at "business people who will be working with data scientists, managing data science-oriented projects, or investing in data science ventures" (p xiii), the book never points out that all analytic techniques make assumptions and that the data scientist needs to be questioned about that (when they don't mention it upfront) and questioned about what happens when assumptions are violated; in addition, many, maybe most, techniques have biases and these are never mentioned either; there is also no discussion of bootstrap (the authors use cross-validation instead thus, generally, wasting information) or of external validation and no warnings about what to beware of when using surrogates; at a lower level, the book is generally readable and generally well-informed but needs to be supplemented with something that covers how to, at least, question the technical people about assumptions and biases
★ ★ ★ ★ ☆
juliet hougland
First 2 chapters contains nothing. The rest chapters gets your mind straight on a few classical models regarding how the work, how to evaluate the models, and some common pitfalls. Fantastic material from Chapter 2.
This book does not contain matereials related to Big Data
This book does not contain matereials related to Big Data
You May Ask Yourself An Introduction to Thinking Like a Sociologist :: Five Principles for Keeping Life in Perspective - You Can Be Happy No Matter What :: A Rosemary Beach Novel (The Rosemary Beach Series) :: Sometimes It Lasts (Sea Breeze) :: An Introduction to Thinking Like a Sociologist (Second Edition)
★ ★ ★ ☆ ☆
alan pursell
As others have noted, this book gives a nice intuitive overview of data mining; however, the Kindle version does not have a table of contents. This is inexcusable for a book of this nature that is just as likely to be used as a reference than read cover to cover. I will note that the iBook version, while much more expensive, does include a table of contents.
★ ★ ★ ☆ ☆
angel walk
I must question the many positive reviews of this book. It's advertised as being based on an NYU/Stern MBA course. If this passes for MBA-level Data Science, count me as shocked. It's more in line with what one would find in an entry-level undergraduate course - many definitions and painful repetitions. Am still searching for a Data Science book with a little heft, and I consider myself a layman.
★ ★ ★ ★ ★
lepton
This book tells you how to **think** from the angle of data when you make decisions. I have read so many data mining books. They often claim themselves practical simply because they provide examples in addition to the technical details. Well, this statement can be seriously misleading since no one is going to solve the same problem as the one in the book. Without a good explanation on the intuition underlying the technique, it is hard to make true links with examples and eventually even harder, if not possible, to extend what you read to what you need to solve in real applications.
This book does an excellent job in this perspective. All the fundamental DM ideas (although there are so many different DM algorithms, they are all variations of only a few fundamental ideas) are explained by almost plain words illustrating human's thinking process. You will feel all the DM methods are familiar even though you have never learned them because they are presented just as a codification of rational thinking in everyday life. Once the intuition is uncovered this well, the examples in the book look so natural and you get a way to start doing your own DM tasks.
It can be your first DM book or an insightful book worth revisiting from time to time. I, as a DM educator, enjoy reading the book and learn a lot not only the insights but also how to transmit DM knowledge effectively.
I love this book!
This book does an excellent job in this perspective. All the fundamental DM ideas (although there are so many different DM algorithms, they are all variations of only a few fundamental ideas) are explained by almost plain words illustrating human's thinking process. You will feel all the DM methods are familiar even though you have never learned them because they are presented just as a codification of rational thinking in everyday life. Once the intuition is uncovered this well, the examples in the book look so natural and you get a way to start doing your own DM tasks.
It can be your first DM book or an insightful book worth revisiting from time to time. I, as a DM educator, enjoy reading the book and learn a lot not only the insights but also how to transmit DM knowledge effectively.
I love this book!
★ ★ ★ ★ ★
jacqueline silvester
Foster Provost and Tom Fawcett have set out to write the go-to reference on Big Data. 'Data Science for Business', what you need to know about Data Mining and Data-Analytic Thinking, published by O'Reilly Media.
They have produced an authoritative book that is both a pathfinder and a lighthouse. It is a long, clearly-written book that shows what can be done using Big Data, where to go and what techniques to use to get it done, and what to watch out for.
Thank you for writing this book. The authors and their many references are already established and respected. The book brings the issues and their business applications together in one essential place. Already in just 1 month since release (25th July 2013) the eBook has gathered praise quotes from a dozen industry names. I am honoured to receive a complimentary review copy.
So to add to the recommendations, I pitch my review slightly differently: Who in business should buy this book? What does this book add to what we are already doing in business with Data and Data Mining?
On first reading, if you work in analysis, IT, Business Intelligence, Management Reporting, Marketing or SEO, I guarantee your reaction at some point will be 'I do that too'.
For me the 'Aha!' realisation came a few pages into chapter 2. The authors discuss database searches for the most profitable items in a business. All businesses do that every day! But not always in the way the academics think.
The book surprised me in covering a broader range of topics than I previously considered were Data Science. Here are some great success stories to illustrate what data science is. Buy the book to see how these things really work and how the leading companies are applying themselves to these challenges. These studies border on the commercially sensitive.
- How a supermarket can use their sales analysis to predict when people are expecting a baby, and so gain an advantage by making offers before their competitors.
- How advertisers use Facebook Likes to profile and segment their audience
- How Netflix make their movie recommendations
- How to compare web pages for plagiarism
- How to tell how far away a customer is from their mobile app
Chapter 10 talks about text analysis. In contrast to most of the book, I would say here that small and medium sized businesses are ahead of Google and the academics. While the search engines refine their algorithms to extract news and meaning from bare text, there is whole industry sector manipulating the source data to fool the algorithms and keep one step ahead: it is called Search Engine Optimisation.
If you are just starting out in using Big Data for your business decisions, you need to know the importance of Maths. In particular there are 2 challenges in the mathematics that underpin Data Science that I should warn you about even if you do not read the book:
* One is causation and correlation. When you find the beer-buying customers are also the nappy-buying customers, that is just the first step towards some very careful thinking before you draw any conclusions about which is cause and which is effect and how you might adjust your marketing or product mix to assist your customers accordingly
* The other is what is now called 'Overfitting'. Gaze hard enough and you will find trends in data just like you can find shapes in clouds or patterns on the back of your eyelids. If you search too hard through too much data, you invalidate correlation co-efficients and confidence calculations. Or to put it another way, every cloud looks like something.
A great book. For everyone who can still manage their high-school level maths, I recommend you buy this book. For everyone else, I recommend you be aware of the book and the issues within it and get it on the corporate bookshelf. For myself I look forward to checking back regularly for future editions as the science develops. Five stars.
They have produced an authoritative book that is both a pathfinder and a lighthouse. It is a long, clearly-written book that shows what can be done using Big Data, where to go and what techniques to use to get it done, and what to watch out for.
Thank you for writing this book. The authors and their many references are already established and respected. The book brings the issues and their business applications together in one essential place. Already in just 1 month since release (25th July 2013) the eBook has gathered praise quotes from a dozen industry names. I am honoured to receive a complimentary review copy.
So to add to the recommendations, I pitch my review slightly differently: Who in business should buy this book? What does this book add to what we are already doing in business with Data and Data Mining?
On first reading, if you work in analysis, IT, Business Intelligence, Management Reporting, Marketing or SEO, I guarantee your reaction at some point will be 'I do that too'.
For me the 'Aha!' realisation came a few pages into chapter 2. The authors discuss database searches for the most profitable items in a business. All businesses do that every day! But not always in the way the academics think.
The book surprised me in covering a broader range of topics than I previously considered were Data Science. Here are some great success stories to illustrate what data science is. Buy the book to see how these things really work and how the leading companies are applying themselves to these challenges. These studies border on the commercially sensitive.
- How a supermarket can use their sales analysis to predict when people are expecting a baby, and so gain an advantage by making offers before their competitors.
- How advertisers use Facebook Likes to profile and segment their audience
- How Netflix make their movie recommendations
- How to compare web pages for plagiarism
- How to tell how far away a customer is from their mobile app
Chapter 10 talks about text analysis. In contrast to most of the book, I would say here that small and medium sized businesses are ahead of Google and the academics. While the search engines refine their algorithms to extract news and meaning from bare text, there is whole industry sector manipulating the source data to fool the algorithms and keep one step ahead: it is called Search Engine Optimisation.
If you are just starting out in using Big Data for your business decisions, you need to know the importance of Maths. In particular there are 2 challenges in the mathematics that underpin Data Science that I should warn you about even if you do not read the book:
* One is causation and correlation. When you find the beer-buying customers are also the nappy-buying customers, that is just the first step towards some very careful thinking before you draw any conclusions about which is cause and which is effect and how you might adjust your marketing or product mix to assist your customers accordingly
* The other is what is now called 'Overfitting'. Gaze hard enough and you will find trends in data just like you can find shapes in clouds or patterns on the back of your eyelids. If you search too hard through too much data, you invalidate correlation co-efficients and confidence calculations. Or to put it another way, every cloud looks like something.
A great book. For everyone who can still manage their high-school level maths, I recommend you buy this book. For everyone else, I recommend you be aware of the book and the issues within it and get it on the corporate bookshelf. For myself I look forward to checking back regularly for future editions as the science develops. Five stars.
★ ★ ★ ★ ★
joe lanman
As the authors discuss in the preface to this text, the content presented here provides a conceptual foundation for many well-known data mining algorithms, and is therefore not about algorithms or a replacement for a book about algorithms. "We believe there is a relatively small set of fundamental concepts or principles that underlie techniques for extracting useful knowledge from data." While this book does contain significant technical content, the conceptual approach that the authors take revolves around (1) how data science fits in the organization and the competitive landscape, (2) ways of thinking data-analytically, which help identify appropriate data and consider appropriate methods, and (3) discussions on extracting the knowledge from data that undergirds the vast array of data science tasks and algorithms.
Content is broken down into 14 chapters, followed by two appendixes which outline factors to consider when assessing potential data mining projects and provide another sample proposal beyond what was presented in Chapter 13 ("Data Science and Business Strategy"). After providing an introduction to data-analytic thinking, the authors present discussions on the following topics: the data mining process, supervised versus unsupervised data mining, identifying informative attributes, segmenting data by progressive attribute selection, fitting a model to data, overfitting and its avoidance, similarity, neighbors, and clusters, model evaluation, model performance, evidence and probabilities, representing and mining text, and analytical engineering, followed by some additional tasks and techniques which build on the foundation presented in earlier chapters, and a discussion of data science and strategy.
The content that the authors present will likely be weighty for many potential readers. While I do agree with the authors that the math is kept to a minimum, this weightiness will likely be due to the number of topics that are discussed as well as the detail of many of the discussions. In short, most will not find this book a short read, although I did find it curious how many book reviews were written so soon after the publish date. Readers who do not have time to read the entire text but are interested in this space might at minimum be advised to read Chapter 1 ("Introduction: Data-Analytic Thinking"), Chapter 2 ("Business Problems and Data Science Solutions"), and Chapter 13 ("Data Science and Business Strategy"), followed by the appendixes, before moving forward to the remaining chapters where a bulk of the material is presented.
As a consultant architect, I especially appreciated the discussion in Chapter 1 ("Introduction: Data-Analytic Thinking") on where data science fits in the context of other data-related processes in the organization, as well as its relationship with Big Data (it is refreshing to read material that presents the correct definition of the term, unlike some other publications in this space). In addition, I enjoyed the discussions in Chapter 7 ("Decision Analytic Thinking I: What Is a Good Model?") and Chapter 11 ("Decision Analytic Thinking II: Toward Analytical Engineering"). The other chapters that I especially appreciate include Chapter 3 ("Introduction to Predictive Modeling: From Correlation to Supervised Segmentation"), Chapter 4 ("Fitting a Model to Data"), Chapter 5 ("Overfitting and its Avoidance"), and Chapter 6 ("Similarity, Neighbors, and Clusters"). And now that I have listed these favorites, I realize that together they comprise half the text.
While I understand that this book is based on an MBA course that Provost taught at NYU over the past ten years, and "The Wall Street Journal" discussed just today that analytics is starting to be increasingly prevalent in MBA coursework, I am impressed at the level of detail in some of the discussions that the authors present, even though the varying language used in some of the segments seems to point to several different authors. As a visual thinker, however, it is the abundant level of diagrams that continued to grab my attention and bring me to understand them in light of the textual component of the discussions. Recommended reading for anyone new to data science or anyone concentrating in one area of the field that seeks better understanding of the big picture.
Content is broken down into 14 chapters, followed by two appendixes which outline factors to consider when assessing potential data mining projects and provide another sample proposal beyond what was presented in Chapter 13 ("Data Science and Business Strategy"). After providing an introduction to data-analytic thinking, the authors present discussions on the following topics: the data mining process, supervised versus unsupervised data mining, identifying informative attributes, segmenting data by progressive attribute selection, fitting a model to data, overfitting and its avoidance, similarity, neighbors, and clusters, model evaluation, model performance, evidence and probabilities, representing and mining text, and analytical engineering, followed by some additional tasks and techniques which build on the foundation presented in earlier chapters, and a discussion of data science and strategy.
The content that the authors present will likely be weighty for many potential readers. While I do agree with the authors that the math is kept to a minimum, this weightiness will likely be due to the number of topics that are discussed as well as the detail of many of the discussions. In short, most will not find this book a short read, although I did find it curious how many book reviews were written so soon after the publish date. Readers who do not have time to read the entire text but are interested in this space might at minimum be advised to read Chapter 1 ("Introduction: Data-Analytic Thinking"), Chapter 2 ("Business Problems and Data Science Solutions"), and Chapter 13 ("Data Science and Business Strategy"), followed by the appendixes, before moving forward to the remaining chapters where a bulk of the material is presented.
As a consultant architect, I especially appreciated the discussion in Chapter 1 ("Introduction: Data-Analytic Thinking") on where data science fits in the context of other data-related processes in the organization, as well as its relationship with Big Data (it is refreshing to read material that presents the correct definition of the term, unlike some other publications in this space). In addition, I enjoyed the discussions in Chapter 7 ("Decision Analytic Thinking I: What Is a Good Model?") and Chapter 11 ("Decision Analytic Thinking II: Toward Analytical Engineering"). The other chapters that I especially appreciate include Chapter 3 ("Introduction to Predictive Modeling: From Correlation to Supervised Segmentation"), Chapter 4 ("Fitting a Model to Data"), Chapter 5 ("Overfitting and its Avoidance"), and Chapter 6 ("Similarity, Neighbors, and Clusters"). And now that I have listed these favorites, I realize that together they comprise half the text.
While I understand that this book is based on an MBA course that Provost taught at NYU over the past ten years, and "The Wall Street Journal" discussed just today that analytics is starting to be increasingly prevalent in MBA coursework, I am impressed at the level of detail in some of the discussions that the authors present, even though the varying language used in some of the segments seems to point to several different authors. As a visual thinker, however, it is the abundant level of diagrams that continued to grab my attention and bring me to understand them in light of the textual component of the discussions. Recommended reading for anyone new to data science or anyone concentrating in one area of the field that seeks better understanding of the big picture.
★ ★ ★ ★ ★
luthien
As Foster Provost and Tom Fawcett explain in the Preface, they examine concepts that fall within one of three types:
"1. Concepts about how data science fits into the organization and the competitive landscape, including ways to attract, structure, and nurture data science teams; ways for think about how data science leads to competitive advantage; and tactical concepts for doing well with data science projects.
2. General ways of thinking data, analytically. These help in identifying appropriate data and consider appropriate methods. The concepts include the [begin italics] data mining process [end italics] as well as the collection of different [begin italics] high-level data mining tasks. [end italics]
3. General concepts for actually extracting knowledge from data, which undergird the vast array of data science tasks and their algorithms."
There you have the nature and extent of the WHAT on which the information, insights, and counsel focus. Provost and Fawcett devote most of their attention to explaining HOW to apply these concepts to achieve high-impact data mining driven by data-analytic thinking. I share their belief "that explaining data science around such fundamental concepts not only aids the reader, it also facilitates communication between and among business stakeholders and data scientists. It provides a shared vocabulary and enables both parties [data scientists and non-data scientists such as I] to understand each other better. The shared concepts lead to deeper discussions that may uncover critical issues otherwise missed."
These are among the dozens of business subjects and issues of special interest and value to me, also listed to indicate the scope of Provost and Fawcett's coverage.
o From Big Data 1.0 to Big Data 2.0 (Pages 9-13)
o From Business Problems to Data Mining Tasks(19-23)
o The Data Mining Process. (26-34)
o Other Analytics Techniques and Technologies (Pages 35-41 and 187-208)
o Selecting Informative Attributes (49-56)
o Supervised Segmentation with Tree-Structured Models (62-67)
o Class Probability Estimation and Logistic "Regression" (97-100)
o Overfitting (113-119)
Note: This is a tendency to tailor models to the training data.
o Correlation of Similarity and Distance (142-144)
o Some Important Technical Details Relating to Similarities and Neighbors (157-161)
o Stepping Back: Solving a Business Problem Versus Data Exploration (183-185)
o A Key Analytical Framework: Expected Value (194-204)
o A Model of Evidence Lift" (244-246)
o Decision Analytic Thinking II: Toward Analytic Engineering (279-289)
o Co-occurrences and Associations: Finding Items That Go Together 292-298)
o Bias, Variance, and Ensemble Methods 308-311)
o Sustaining Competitive Advantage with Data Science (318-323)
As I worked my way through the book a second time, in preparation to compose this review, I was again reminded of comments by Eric Schmidt, executive chairman of Google: "From the dawn of civilization until 2003, mankind generated five exabytes of data. Now we produce five exabytes every two days...and the pace is accelerating." Correspondingly, the challenges that this process of data accumulation creates will become even greater. Provost and Fawcett wrote this book for those who must manage this process but also to assist the efforts of instructors who are now preparing them to do so.
"1. Concepts about how data science fits into the organization and the competitive landscape, including ways to attract, structure, and nurture data science teams; ways for think about how data science leads to competitive advantage; and tactical concepts for doing well with data science projects.
2. General ways of thinking data, analytically. These help in identifying appropriate data and consider appropriate methods. The concepts include the [begin italics] data mining process [end italics] as well as the collection of different [begin italics] high-level data mining tasks. [end italics]
3. General concepts for actually extracting knowledge from data, which undergird the vast array of data science tasks and their algorithms."
There you have the nature and extent of the WHAT on which the information, insights, and counsel focus. Provost and Fawcett devote most of their attention to explaining HOW to apply these concepts to achieve high-impact data mining driven by data-analytic thinking. I share their belief "that explaining data science around such fundamental concepts not only aids the reader, it also facilitates communication between and among business stakeholders and data scientists. It provides a shared vocabulary and enables both parties [data scientists and non-data scientists such as I] to understand each other better. The shared concepts lead to deeper discussions that may uncover critical issues otherwise missed."
These are among the dozens of business subjects and issues of special interest and value to me, also listed to indicate the scope of Provost and Fawcett's coverage.
o From Big Data 1.0 to Big Data 2.0 (Pages 9-13)
o From Business Problems to Data Mining Tasks(19-23)
o The Data Mining Process. (26-34)
o Other Analytics Techniques and Technologies (Pages 35-41 and 187-208)
o Selecting Informative Attributes (49-56)
o Supervised Segmentation with Tree-Structured Models (62-67)
o Class Probability Estimation and Logistic "Regression" (97-100)
o Overfitting (113-119)
Note: This is a tendency to tailor models to the training data.
o Correlation of Similarity and Distance (142-144)
o Some Important Technical Details Relating to Similarities and Neighbors (157-161)
o Stepping Back: Solving a Business Problem Versus Data Exploration (183-185)
o A Key Analytical Framework: Expected Value (194-204)
o A Model of Evidence Lift" (244-246)
o Decision Analytic Thinking II: Toward Analytic Engineering (279-289)
o Co-occurrences and Associations: Finding Items That Go Together 292-298)
o Bias, Variance, and Ensemble Methods 308-311)
o Sustaining Competitive Advantage with Data Science (318-323)
As I worked my way through the book a second time, in preparation to compose this review, I was again reminded of comments by Eric Schmidt, executive chairman of Google: "From the dawn of civilization until 2003, mankind generated five exabytes of data. Now we produce five exabytes every two days...and the pace is accelerating." Correspondingly, the challenges that this process of data accumulation creates will become even greater. Provost and Fawcett wrote this book for those who must manage this process but also to assist the efforts of instructors who are now preparing them to do so.
★ ★ ★ ★ ★
jen bubnash askey
Most books on Data Science, Machine Learning and "Big Data" either oversimplify the underlying science and mathematical principles or are too deep to be understood by non-mathematical business professionals and those who seek to use these increasingly important decision making tools. This primer for business professionals and others who wish to apply Data Science to practical decision making precisely hits the ideal middle ground explaining how these tools can be used in practical heuristic decision making; it presents the minimally required mathematical principles in the simplest possible form without being overly simplistic and obscuring the working principles. It employs clear graphics and a simplified mathematical presentation of necessary formulas to the best effect in illustrating the tools of decision making. It was written as a collaboration between a data scientist who teaches MBA's and business professionals, and a PhD researcher in Machine Learning who has applied these techniques in leading technology firms.
In my opinion it is the best book on Data Science and Big Data for a professional understanding by business analysts and managers who must apply these techniques in the practical world. One intention omission that is best filled by another handbook is on the data processing tools that are applied in
exploiting these well explained principles: NoSql Databases, Hadoop, Scripting Languages such as Python, and Statistical Shells such as Weka, Orange, R and Matlab.
--Ira Laefsky MS Engineering, MBA Information Technology Consultant and Physiological Computing Researcher
formerly on the Senior Consulting Staff of Arthur D. Little, Inc. and Digital Equipment Corporation
In my opinion it is the best book on Data Science and Big Data for a professional understanding by business analysts and managers who must apply these techniques in the practical world. One intention omission that is best filled by another handbook is on the data processing tools that are applied in
exploiting these well explained principles: NoSql Databases, Hadoop, Scripting Languages such as Python, and Statistical Shells such as Weka, Orange, R and Matlab.
--Ira Laefsky MS Engineering, MBA Information Technology Consultant and Physiological Computing Researcher
formerly on the Senior Consulting Staff of Arthur D. Little, Inc. and Digital Equipment Corporation
★ ★ ★ ★ ★
brandon burrup
Excellent! Data Science for Business is an extremely well written practitioner's guide. The concepts and methodologies found inside ARE NOT EASY TO FIND in one comprehensive, comprehendible, and no-nonsense book. I received the book initially as part of my Master of Science in Business Analytics curriculum, and still, I purchased the eBook to have with me and use for my work. In fact, the principles and techniques covered in the book reshaped my understanding of big data business analytics and exactly how to do it and do it right.
★ ★ ★ ★ ★
cindy o
I bought this book because it seemed to address my current situation well. I'm a software developer with years of experience, but never got on the 'big data' bandwagon. As it happens I have an MBA, and was looking for a 'big picture' resource rather than a technical manual. I wasn't disappointed, the book is fairly easy to read with good points to remember. I admit I got bogged down in the math, but as long as I know it's there and know what it can do, I can return to it once I have an application for it. Also really helpful was a section on how to write business proposals for data analysis, which is an often overlooked business function. Every project starts with a proposal giving a cost/benefits analysis, so that drew my attention most of all. You have to explain to a paying client "why" as well as "how", so that was nice to read.
So this is a great book for in-betweeners like me. I don't need a tutorial on how to program, but I'm not so well versed in math that nuances of statistics are obvious to me. It's a book I'll keep handy in situations where I'll have an opportunity to make some money with large data sets, and this book will brief me on what I need to know to move forward.
-Derrick
So this is a great book for in-betweeners like me. I don't need a tutorial on how to program, but I'm not so well versed in math that nuances of statistics are obvious to me. It's a book I'll keep handy in situations where I'll have an opportunity to make some money with large data sets, and this book will brief me on what I need to know to move forward.
-Derrick
★ ★ ★ ★ ★
kirstie mayes
This book is a great introduction to data science. It works with people who don't have all the math background. But it will also cover some of the math topics (with a note that they are about to go into a math based section, and you can skip it if you aren't ready). This is a must have for managers, people in business, and people just interested in learning more about Data Science.
If you are planning to know enough for your business to be better, this is your book. If you are planning on delving into the depths, you will need to also pursue things like linear algebra and other advanced math topics, but this book will help you with each step you can follow on with. Good luck on your journey!
If you are planning to know enough for your business to be better, this is your book. If you are planning on delving into the depths, you will need to also pursue things like linear algebra and other advanced math topics, but this book will help you with each step you can follow on with. Good luck on your journey!
★ ★ ★ ★ ★
inmi
This is an excellent introduction to Data Science for the person who wants to gain a good understanding of the subject and what it can do for business. The authors’ language is straightforward and they have attempted to simplify statistical nomenclature to avoid losing their less statistically qualified readers.
Provost and Fawcett have put together a very accessible guide that explains what Data Science is and what it is not. Their work is realistic and practical. The book presents the theory behind the subject and includes practical applications that enhance the reader’s understanding. Provost and Fawcett are excellent at swiping away myths on the subject, myths that some commercial promoters of the subject may like to maintain. The power of Data Science is amazing enough without reliance on myth. The authors clearly state that Data Science is not the panacea for all ills, and that it cannot succeed without the understanding of people.
Not only does this book deal with the theoretical and technical concepts of the subject, but it discusses how the discipline can work within an organisation and with such issues as data privacy.
It is seldom one finds a book that is so clear and comprehensive.
Provost and Fawcett have put together a very accessible guide that explains what Data Science is and what it is not. Their work is realistic and practical. The book presents the theory behind the subject and includes practical applications that enhance the reader’s understanding. Provost and Fawcett are excellent at swiping away myths on the subject, myths that some commercial promoters of the subject may like to maintain. The power of Data Science is amazing enough without reliance on myth. The authors clearly state that Data Science is not the panacea for all ills, and that it cannot succeed without the understanding of people.
Not only does this book deal with the theoretical and technical concepts of the subject, but it discusses how the discipline can work within an organisation and with such issues as data privacy.
It is seldom one finds a book that is so clear and comprehensive.
★ ★ ★ ☆ ☆
abatage
"Data Science", "Data Mining" and "Predictive Analytics" are some of the terms of recent vintage that define the application of modern mathematics, enabled by twenty-first century computing power, to identify patterns in the staggering quantities of data that can be so easily acquired and accessed. This new technology has such important ramifications in areas ranging from market behaviour to cyber security that even laymen need to have an understanding of its far reaching implications. One recent effort to make these ideas intelligible to the non-expert, the rather puerile "Predictive Analytics" by Eric Siegel, failed miserably. This book comes much closer to the mark.
With a readable, almost conversational, style Provost and Fawcett describe some of the fundamental notions in data science, casually discussing such standard topics as supervised and unsupervised learning, clustering, regression, linear discriminants, model building and even a pseudo introduction to Support Vector Machines. There is also a chapter on the danger of over fitting, a common malady afflicting those new to machine learning. Of course, the authors' stated desire to avoid any genuine maths renders some of the descriptions opaque and even misleading. Nevertheless, the alert layman will come away with a decent familiarity with some of the concepts and methods employed in this rapidly evolving technology.
With a readable, almost conversational, style Provost and Fawcett describe some of the fundamental notions in data science, casually discussing such standard topics as supervised and unsupervised learning, clustering, regression, linear discriminants, model building and even a pseudo introduction to Support Vector Machines. There is also a chapter on the danger of over fitting, a common malady afflicting those new to machine learning. Of course, the authors' stated desire to avoid any genuine maths renders some of the descriptions opaque and even misleading. Nevertheless, the alert layman will come away with a decent familiarity with some of the concepts and methods employed in this rapidly evolving technology.
★ ★ ★ ★ ★
lotzastitches
Companies to compete today are seeking new ways to dramatically improve operational efficiency, increase revenues, rapidly develop and bring innovative products to market. As a result, data science principles and data mining techniques are being expanded and applied at an ever increasing rate to support these strategies. Almost every industry is investing in the exploitation of data to find any competitive advantage it can.
To help those less conversant on these new tools, authors Foster Provost and Tom Fawcett have produced "Data Science for Business." This will be a particularly useful book for business people who will be working in the field, business people (like me) who just want a basic understanding of how it can be and should be used, developers who will be implementing solutions, candidates for data science jobs, and, of course, their hiring managers. The book is a product of Provost and Fawcett's applying data science to real business problems for more than two decades. It was introduced by Provost as a textbook for New York University's Stern School in 2005.
This is not a book about algorithms or an instructional manual on how to find data solutions for particular problems but rather an introduction to a small set of fundamental concepts that underlie techniques for extracting useful knowledge from data. These provide the foundation and building blocks for all algorithms.
While the authors claim that the reader does not need a "sophisticated mathematical background," the reader will need more than basic math to grasp the underlying concepts. Remember that this text is used as a college text book so do not expect a quick and easy read...the material is very technical as the authors intend to impart a significant understanding of data science.
The primary goals of this book are to help the reader view business problems from a data perspective and to understand principles of extracting useful knowledge from data. A data perspective provides structure and principles; an objective framework to systemically analyze business problems. In today's world, it has become increasingly important to, at the very least, understand the basics of data science, even if you never intend to use it yourself. Data analytic thinking is the key to business in the 21st Century.
To help those less conversant on these new tools, authors Foster Provost and Tom Fawcett have produced "Data Science for Business." This will be a particularly useful book for business people who will be working in the field, business people (like me) who just want a basic understanding of how it can be and should be used, developers who will be implementing solutions, candidates for data science jobs, and, of course, their hiring managers. The book is a product of Provost and Fawcett's applying data science to real business problems for more than two decades. It was introduced by Provost as a textbook for New York University's Stern School in 2005.
This is not a book about algorithms or an instructional manual on how to find data solutions for particular problems but rather an introduction to a small set of fundamental concepts that underlie techniques for extracting useful knowledge from data. These provide the foundation and building blocks for all algorithms.
While the authors claim that the reader does not need a "sophisticated mathematical background," the reader will need more than basic math to grasp the underlying concepts. Remember that this text is used as a college text book so do not expect a quick and easy read...the material is very technical as the authors intend to impart a significant understanding of data science.
The primary goals of this book are to help the reader view business problems from a data perspective and to understand principles of extracting useful knowledge from data. A data perspective provides structure and principles; an objective framework to systemically analyze business problems. In today's world, it has become increasingly important to, at the very least, understand the basics of data science, even if you never intend to use it yourself. Data analytic thinking is the key to business in the 21st Century.
★ ★ ★ ★ ★
barbara falkiner
I teach Data Mining and Business Analytics courses. I recently started using this book as a textbook for an introductory level, MBA, data science course. I find the book to be an exceptionally good textbook. Unlike many other books in this field that focus on the different Data Mining tasks and the specific algorithms used to implement them - this book's focus is on the basic concepts of data science. The authors did a very good job in first distilling, and then explaining and demonstrating many important fundamental concepts of data science. This is an important added value for an introductory level book, and which renders the book also useful for Data Mining professionals who could benefit from the collection of formalized concepts and the related discussion. Moreover, the book can extend an excellent footing for those wishing to gradually get into the field of data science.
I would definitely recommend this book to both people novice to data science as well as to professionals. Notwithstanding, it is important to note that the book generally avoids mathematical depth when detailing various algorithms/methods. I expect that this has been done intentionally to keep the discussion clear for as wide an audience as possible. Those seeking to learn the specifics of various algorithms can find this additional information in various advanced-level textbooks or academic papers.
Finally, I would like to specify two chapters that I liked most. The first is the introductory chapter that "organizes" and puts into place various terms (and sometimes buzzwords) related to this field (E.g., "Big data", Data Science, etc). The second is the chapter titled "Decision Analytic Thinking II: Toward Analytical Engineering" which uses the "expected value framework" to provide an excellent demonstration of the careful thinking required to state a real life business problem as a sequence of Data Mining tasks.
I would definitely recommend this book to both people novice to data science as well as to professionals. Notwithstanding, it is important to note that the book generally avoids mathematical depth when detailing various algorithms/methods. I expect that this has been done intentionally to keep the discussion clear for as wide an audience as possible. Those seeking to learn the specifics of various algorithms can find this additional information in various advanced-level textbooks or academic papers.
Finally, I would like to specify two chapters that I liked most. The first is the introductory chapter that "organizes" and puts into place various terms (and sometimes buzzwords) related to this field (E.g., "Big data", Data Science, etc). The second is the chapter titled "Decision Analytic Thinking II: Toward Analytical Engineering" which uses the "expected value framework" to provide an excellent demonstration of the careful thinking required to state a real life business problem as a sequence of Data Mining tasks.
★ ★ ★ ★ ★
ashrith
Data Science for Business by Foster Provost and Tom Fawcett (O'Reilly Media) is a book that makes a phenomenal job teaching the fundamental concepts of Data Science (a.k.a. Data Analysis and Data Mining). Foster Provost and Tom Fawcett explain in plain English, clear examples and beginner-level math the processes surrounding Data Science and the basics of its algorithms.
The authors go over the various steps of the CRISP method using situations found in the real world such as Customer Churn and Online Advertising. The most common data analysis models are reviewed and explained in detail such as Clustering, Decision Trees and Support Vector Machines. Extensive explanation is given to the difference between supervised and unsupervised methods. Even if you use software tools that create those models, this book will help you understand how to use/test them correctly and how to avoid over-fitting.
Multiple examples are given in each chapter and most of the math is visually aided with graphs. The authors explain step by step any equation presented in the book. A notable example is how the authors show how the different parts of the Bayes' Rule equation come together in chapter 9. There are also special Math-intensive sections that business managers might skip, but software developers and future data scientist need to examine closely.
I would recommend this book to any DBA or Developer looking for an useful introduction to Data Science. For a practical application of the concepts in the book, I recommend Data Analysis Using SQL and Excel by Gordon Linoff after reading Data Science for Business. As a SQL Server DBA, I will apply the concepts I learned with the book to SQL Server Analysis Services.
The authors go over the various steps of the CRISP method using situations found in the real world such as Customer Churn and Online Advertising. The most common data analysis models are reviewed and explained in detail such as Clustering, Decision Trees and Support Vector Machines. Extensive explanation is given to the difference between supervised and unsupervised methods. Even if you use software tools that create those models, this book will help you understand how to use/test them correctly and how to avoid over-fitting.
Multiple examples are given in each chapter and most of the math is visually aided with graphs. The authors explain step by step any equation presented in the book. A notable example is how the authors show how the different parts of the Bayes' Rule equation come together in chapter 9. There are also special Math-intensive sections that business managers might skip, but software developers and future data scientist need to examine closely.
I would recommend this book to any DBA or Developer looking for an useful introduction to Data Science. For a practical application of the concepts in the book, I recommend Data Analysis Using SQL and Excel by Gordon Linoff after reading Data Science for Business. As a SQL Server DBA, I will apply the concepts I learned with the book to SQL Server Analysis Services.
★ ★ ★ ★ ☆
julia vaughn
Data Science for Business is one of several data science titles from O'Reilly. It is probably the most comprehensive in terms of theory. That said, it feels a bit too comprehensive, including everything from statistical methods to research proposals to how to talk to your data people. One thing it doesn't get into though is the actual technology. It is assumed that your data experts will figure that out and you'll ask technology questions at the meta level. It's an approach that would make more sense if you spent more time understanding results at the meta level too. As a result I would suggest this otherwise comprehensive tome needs an accompanying guide to data science technology.
If you are an executive with low level experience doing data science, e.g. with Excel sheets for company specific data, this is a good guide to thinking seriously about higher level stuff. But if you are truly curious about what this data science stuff is all about, I would recommend starting with a different O'Reilly title, Doing Data Science, which provides more of a ground level approach to what you can do with data science and how.
If you are an executive with low level experience doing data science, e.g. with Excel sheets for company specific data, this is a good guide to thinking seriously about higher level stuff. But if you are truly curious about what this data science stuff is all about, I would recommend starting with a different O'Reilly title, Doing Data Science, which provides more of a ground level approach to what you can do with data science and how.
★ ★ ★ ☆ ☆
rhiann
This is a pretty decent overview of data science. However, it is a little too technical for me to feel comfortable giving to my non-technical manager. And it is not quite technical enough to actually allow you to implement any of the techniques it mentions. It's not bad, but it doesn't really stand on it's own.
★ ★ ★ ★ ☆
hadis malekie
If you're thinking of analyzing large datasets for common business applications (e.g. targeted marketing), this book will give you everything you need to know and then some. It not only gives an overview of the most popular techniques for prediction and categorization, but also highlights some of the subtler technical issues involved (overfitting, choosing an appropriate loss function) as well as higher-level concerns (privacy, comprehensibility). My only quibble is that some of the visualizations shown in the book are not adequately explained.
★ ★ ★ ★ ★
caroline ferguson
I work in an industry that everyone loves to throw out the word big data. I find in my work place it can mean any thing from a large spreadsheet to actual data warehouses. I found this book very helpful in learning about large data and what the true potential data may bring.
I liked the book walked the line between tech and business speak. I want to share this book with my coworkers. An excellent read, that takes away many misconceptions. This book is helpful bridging the gap between IT and business.
I liked the book walked the line between tech and business speak. I want to share this book with my coworkers. An excellent read, that takes away many misconceptions. This book is helpful bridging the gap between IT and business.
★ ★ ★ ★ ★
kady maresh
This is probably one of the most comprehensive books I have ever come across in any field of inquiry. It is clear that the authors took pains to ensure they can engage very different levels of readers into the science. The writing style is absolutely conducive to quick grasp. Many of the topics are quite complex but the style and the method adopted told the reader what to expect, how to receive it as well as what to do with it, in each successive page and chapter. In that way, it is one of the rare non-fictional works that actually tell the story interestingly. While this must be compulsory reading for all aspiring data scientists, analysts and managers, I'd very strongly recommend it (especially the last two chapters) to all corporate executives. In most medium and large sized Operations and Technology projects, the so called 'discovery' phase as well as 'requirements' phase can very significantly be improved with this, not just 'data mining' projects. Get it, read it, refer it and read it again!
★ ★ ★ ★ ★
brennan
This book is a concise, timely, informative and highly readable guide to the burgeoning field of data science and its application to modern business. Through illuminating practical examples (and a healthy dose of humor), Provost and Fawcett manage to (entertainingly) convey the technical guts of modern data mining methods without obfuscating the intuitions behind them.
Refreshingly, the authors do not simply enumerate a hodgepodge of specific data mining algorithms. Rather, the focus is on fundamental concepts that are common across data science applications to business. This affords the reader an appreciation of high-level, data-driven thinking and how it can practically be applied to business problems. The book's blend of concrete business application stories and accompanying expositions of the data mining methods and principles necessary to achieve them renders this a foundational book that should be read by any MBA-type looking to get up to speed with the concepts and technologies that enable data-driven business decision-making.
Refreshingly, the authors do not simply enumerate a hodgepodge of specific data mining algorithms. Rather, the focus is on fundamental concepts that are common across data science applications to business. This affords the reader an appreciation of high-level, data-driven thinking and how it can practically be applied to business problems. The book's blend of concrete business application stories and accompanying expositions of the data mining methods and principles necessary to achieve them renders this a foundational book that should be read by any MBA-type looking to get up to speed with the concepts and technologies that enable data-driven business decision-making.
★ ★ ★ ★ ★
gerrie
Data Science for Business boils up the most important concepts of data science in a manner that is easy to understand and put into practice. After starting several companies, I can tell you from experience that the ability to collect, extract, and analyze data has become one of the most fundamental competitive advantages in today's business environment. Whether you are in marketing, operations, finance, HR or strategy, every decision should be rooted in the fundamentals of data science covered in this book. Provost and Fawcett have built a bridge to help align yourself (and those around you) to this relatively new way of thinking.
I have taken data science courses, gone to numerous data science events, and worked with extremely intelligent data scientists, but I feel like I would have gotten much more out of each had I read this book beforehand. Their book offers a deep dive into the application of data sciences within the business environment without getting caught up on the engineering aspects that make most other DS books difficult to read. I would highly recommend this book to anyone who is interested in learning more about the concept from both a business and technical perspective.
I have taken data science courses, gone to numerous data science events, and worked with extremely intelligent data scientists, but I feel like I would have gotten much more out of each had I read this book beforehand. Their book offers a deep dive into the application of data sciences within the business environment without getting caught up on the engineering aspects that make most other DS books difficult to read. I would highly recommend this book to anyone who is interested in learning more about the concept from both a business and technical perspective.
★ ★ ★ ★ ★
micha szyma ski
I am a business professor from a research institution who teaches business intelligence and data analytics. I have been constantly searching for a good book in the data mining field that covers enough depth of the topics, but can actually be understood by business managers and non-computer-science professionals. Although there are massive amount of data mining and machining learning books in the market, most of them are too theoretical and seriously lack intuition (with regard to how the data mining and statistical models can be connected to the real-world business problems...).
I found this book especially useful and readable because it explains the key concepts and development of data mining and analytical approaches in a very intuitive way. The part I really enjoy is that it tells you a story about your data: why you need a quantitative model for business decision making, how the model is motivated by the business problem, what analytical solutions can effectively fit into the business problem, and how can you evaluate the results to answer your business questions. Moreover, it keeps revisiting the real-world problems and business strategies during the learning of different statistical models. I would strongly recommend this book to anyone in the business world. A must read!
I found this book especially useful and readable because it explains the key concepts and development of data mining and analytical approaches in a very intuitive way. The part I really enjoy is that it tells you a story about your data: why you need a quantitative model for business decision making, how the model is motivated by the business problem, what analytical solutions can effectively fit into the business problem, and how can you evaluate the results to answer your business questions. Moreover, it keeps revisiting the real-world problems and business strategies during the learning of different statistical models. I would strongly recommend this book to anyone in the business world. A must read!
★ ★ ★ ★ ★
julia bowden hall
Provost and Fawcett have given us an insightful, practical, and even entertaining guide to the rapidly evolving field of data science applications for business. As one might expect, it covers concepts, techniques, and equations, but what is especially valuable is their emphasis on the relationship between business understanding and data understanding, and the ways that each must support the other. The authors provide a wide range of examples, from detailed industry case studies to enjoyable analytical forays into the world of single malt scotch whiskeys. I emerged from my reading of DSFB with a greatly enhanced understanding of how a data scientist would approach business problems, how they need to communicate with their business stakeholders, and the strengths and limitations of many different analytical approaches. Highly recommended for those, like myself, that need to interact with data scientists without being one.
★ ★ ★ ★ ★
rana mahmoud
I came across this book when preparing to interview for a sales position at a marketing technology company whose product relies on collecting, interpreting and making data actionable. It took less than a couple of hours to find myself immersed in real, recognizable stories, set as examples of data science's ability to reimagine the synthesis of a problem and present a hidden-from-plain-sight answer. Shortly afterward, I was introduced to the fundamental principles of data science, which expanded my analytical thinking, giving it multidimensionality and an understanding of how to expose a problem's solution, whether in business, the arts, social science, or life itself.
In a world where an ever-increasing amount of actions and interactions are collected and stored in readily available data sets, those who master the analytical thinking to mine them will attain a significant competitive advantage.
In a world where an ever-increasing amount of actions and interactions are collected and stored in readily available data sets, those who master the analytical thinking to mine them will attain a significant competitive advantage.
★ ★ ★ ★ ★
karigriff
Exceptionally well written book! The authors made this book accessible for everyone: from a novice who is just beginning and wants to integrate data science into their current work, to an expert who has formal data science training but wants a nice guide to orient them toward real-world business applications. I fall predominately into the latter category, and found that this book really helped me think more concretely about how the expertise I have amassed over my graduate education in machine learning could be applicable to the challenges faced by businesses. I found it so useful that I am actually citing this book in my dissertation, using it to demonstrate the practical benefits of the statistical machine learning algorithms I have spent my PhD developing. I would recommend this book to anyone: although it does not present any new algorithms, it does however group and layout the core concepts of data science (i.e., data mining and machine learning) algorithms in an extremely clear and coherent manner. As I quickly scan the the many data mining, machine learning and business intelligence books on my shelf, I do not see any that offer a better understanding of the fundamental methods and uses of data science.
★ ★ ★ ★ ☆
christine hernando
As name suggest Data Science for Business is for business people. It is an education book for business people not for technical crowd.
Book explains Data science and data mining concepts in very crisp manner. Those who does not have patience to read whole book should read chapter two. Now if you interested then go ahead. I guess you will.
Book is around 500 pages long which makes it difficult to read but still book has good content for business folks without using arcane jargon.
Book explains Data science and data mining concepts in very crisp manner. Those who does not have patience to read whole book should read chapter two. Now if you interested then go ahead. I guess you will.
Book is around 500 pages long which makes it difficult to read but still book has good content for business folks without using arcane jargon.
★ ★ ★ ★ ★
lareesa
Whether you are coming from a quantitative background or an area with minimum experience in data mining, this book will enable anyone who is interested in Big Data to learn the process of discovering all the necessary tools one needs for successful data exploration and analysis. The authors provide excellent guidance and real world examples throughout each phase of the data mining process.
Additionally, this is not your typical text book, but a fun read. (See similarity measures using the whiskey example). It should be part of your must read book lists because it does not marginalize readers. The writers take you on a journey with such ease, that any reader will revisit it over and over until he/she masters the field.
Additionally, this is not your typical text book, but a fun read. (See similarity measures using the whiskey example). It should be part of your must read book lists because it does not marginalize readers. The writers take you on a journey with such ease, that any reader will revisit it over and over until he/she masters the field.
★ ★ ★ ★ ★
malora70
I read many books on data science / advanced analytics. This one is by far the best. It is specific enough to teach the willing yet uninitiated the foundations of data Science, but at the same very good at portraying the big picture. The entire book is precious, but business executives will agree that Chapter 13 on Data Science, Strategy, and Competitive advantage is the crown jewel. Foreword: the book is very clearly written, but not a read for the beach. You will need to put some time and effort into grasping the concepts. But if you do, you will be handsomely rewarded.
★ ★ ★ ★ ★
romain
Really interesting read. As an experienced data miner, I still learned a lot from reading this book, mainly because of its fascinating state of the art applications (for example I really like the advertising examples) which are tied up nicely with the theory. It provides a unique comprehensible overview of techniques and applications of data science. I started recommending my business partners who work on (any kind of) data to read this book. Also makes for a great textbook for business schools.
★ ★ ★ ★ ★
nathan buchanan
"Data Science for Business" is extremely well written for data scientists. It identifies a small set of fundamental principles underlying data science. By walking you through these fundamentals, you can easily understand many well-known data mining algorithms. A broad set of practical data analytic skills based on real world applications introduced can help you perform data analytics effectively. Overall, it is broad, deep, but not too technical. Readers do not need sophisticated mathematics and statistics background to understand the concepts and methodologies in data science.
★ ★ ★ ★ ★
aimee
I read many books on data science / advanced analytics. This one is by far the best. It is specific enough to teach the willing yet uninitiated the foundations of data Science, but at the same very good at portraying the big picture. The entire book is precious, but business executives will agree that Chapter 13 on Data Science, Strategy, and Competitive advantage is the crown jewel. Foreword: the book is very clearly written, but not a read for the beach. You will need to put some time and effort into grasping the concepts. But if you do, you will be handsomely rewarded.
★ ★ ★ ★ ★
farzan
Really interesting read. As an experienced data miner, I still learned a lot from reading this book, mainly because of its fascinating state of the art applications (for example I really like the advertising examples) which are tied up nicely with the theory. It provides a unique comprehensible overview of techniques and applications of data science. I started recommending my business partners who work on (any kind of) data to read this book. Also makes for a great textbook for business schools.
★ ★ ★ ★ ★
annie h
"Data Science for Business" is extremely well written for data scientists. It identifies a small set of fundamental principles underlying data science. By walking you through these fundamentals, you can easily understand many well-known data mining algorithms. A broad set of practical data analytic skills based on real world applications introduced can help you perform data analytics effectively. Overall, it is broad, deep, but not too technical. Readers do not need sophisticated mathematics and statistics background to understand the concepts and methodologies in data science.
★ ★ ★ ★ ★
jaymes
The book puts many of the popular data mining techniques into a business decision framework by describing the business motivation for the analysis, the steps leading up to and following development of the model, and comparing models that might be used for a particular business decision. It doesn't shrink on the math, providing just enough to really understand what is going on mathematically but not filling the book with lots of equations. I'm a doctoral student in machine learning and was surprised to find that the book, by providing the business setting for the math, helped me to understand some of the mathematical concepts I had previously studied.
This is a really good introductory book that motivates the need for many of the popular methods and soundly explains how they work and why one would want to use them.
This is a really good introductory book that motivates the need for many of the popular methods and soundly explains how they work and why one would want to use them.
★ ★ ★ ★ ★
riham
Coming from a business perspective, i had to learn the math part pretty quickly. This book enables me to do that. It felt like a very well written crash course on the ideas behind the different methods and the math to them.
One note: the link to the KDD cup should be updated to: (...)
Kudos to the author.
One note: the link to the KDD cup should be updated to: (...)
Kudos to the author.
★ ★ ★ ☆ ☆
rose
This is a pretty decent overview of data science. However, it is a little too technical for me to feel comfortable giving to my non-technical manager. And it is not quite technical enough to actually allow you to implement any of the techniques it mentions. It's not bad, but it doesn't really stand on it's own.
★ ★ ★ ★ ★
loni
This is a great book for both beginners and experts. Gives an overview and in depth of the concepts on a broad level. This will help with the theoretical knowledge however the concepts can be implemented using any programming language like python or R.
★ ★ ★ ★ ★
ashish
This book offers right combination of Data Science theory and practice. It is not overly statistical and provides real applications of analytics and data mining. I chose the book to teach data science in my class.
★ ★ ★ ★ ☆
krystal yates
Great introduction to the role of Data Science in Business Analytics applications across any organisation. Avoids math complexities of the Data Science methods and techniques and always keep the focus on business value delivered by a data science solution!
★ ★ ★ ★ ★
sally wentriro
This book is downright awesome. I have read this book twice and I can vouch for every single page of this book to be invaluable. If you are not a statistician but would like to grasp the key fundamentals of data science, look no further. Grab this book and read cover to cover.
★ ★ ★ ★ ★
dunski
This book was an excellent introduction to the world of data science. The writing was very accessible and the examples were clear. I immediately began thinking about how I could analyze data in my company. It provided a great foundation of knowledge and the developing field.
★ ★ ★ ★ ★
josh seol
I use this book to teach an introductory course on data science to a mixed classroom of MS students and advanced undergraduates. The combination of business case studies with intuitive explanations of the math works very well in this context.
★ ☆ ☆ ☆ ☆
michelle munch
Sloppy, careless & badly written book. Someone put together hastily written notes by the authors with no editing at all. The structure is sloppy: some material on techniques, then some on model evaluation, back to techniques, back to model evaluation, etc.; e.g., chapter 5 or 6 presents an elaborate example for text searching, but this is explained in chapter 10 or so; in the same chapter fig. 6-10 explains a 2nd step in a process, the next figure 6-11 explains the first step; other example, chapter on Bayesian techniques: clearly one person wrote the first part clearly explaining notation and formulas, while a different person wrote the section on the "naive Bayesian equation" with far less care. At times the grammar feels as if the book was translated from another language. In the end the book is instructive and I did learn some basic concepts, but I gave it one star because of the extremely poor editing.
★ ★ ★ ★ ★
amber
This is a perfect introduction to Data Science techniques for business professionals.
It is clearly written and engaging, presenting plenty of technical material without making it intimidating to a non-technical audience.
It is clearly written and engaging, presenting plenty of technical material without making it intimidating to a non-technical audience.
★ ★ ☆ ☆ ☆
nora luca
The biggest problem with this book is that it tries to be useful to both the technical side and the business side--and as a result is of limited usefulness to either one. It's also badly organized. The underlying theme seems to be to present data mining in the context of the CRISP-DM methodology, and yet the six phases are not discussed in order. Instead the book jumps around between topics so much it's enough to make you sea sick.
My final criticism is just a pet peeve of mine; you may not care about it---But they constantly use the feminine pronoun as the generic pronoun. (e.g. "A data scientist must make sure she......"). I mean literally every friggin' time over nearly 400 pages. It gets annoying after awhile--especially from 2 ostensibly male authors. I don't know if they are afraid of their colleagues, their wives, or if they're just a couple of standard leftist cuck college professors trying to sound hip. I'd imagine all three are probably true.
My final criticism is just a pet peeve of mine; you may not care about it---But they constantly use the feminine pronoun as the generic pronoun. (e.g. "A data scientist must make sure she......"). I mean literally every friggin' time over nearly 400 pages. It gets annoying after awhile--especially from 2 ostensibly male authors. I don't know if they are afraid of their colleagues, their wives, or if they're just a couple of standard leftist cuck college professors trying to sound hip. I'd imagine all three are probably true.
Please RateWhat You Need to Know about Data Mining and Data-Analytic Thinking
Even thought this not my first time with this material I found this book unclear. Also there were references to websites that were no longer available. ([...]).
I cannot recommend this book.