How Big Data Increases Inequality and Threatens Democracy
ByCathy O%27Neil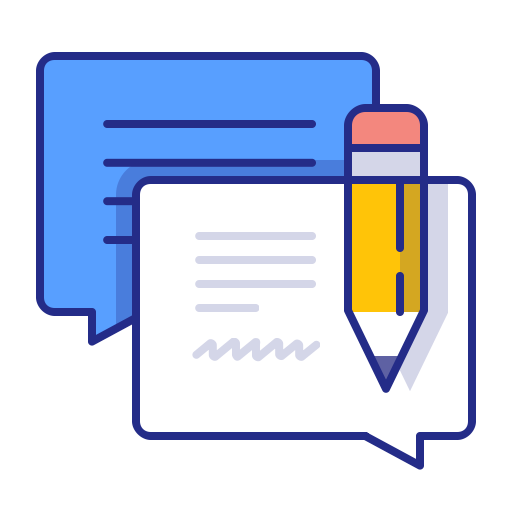
★ ★ ★ ★ ★ | |
★ ★ ★ ★ ☆ | |
★ ★ ★ ☆ ☆ | |
★ ★ ☆ ☆ ☆ | |
★ ☆ ☆ ☆ ☆ |


Check out Audiobooks.com
Readers` Reviews
★ ★ ★ ★ ★
cylia
Just finished this book. I couldn’t put it down. Nearly every free minute was put into completing it. Even had a barbershop conversation about the facts in this book. Definite a read for an ethics class.
★ ★ ★ ★ ★
aghavni
This book is mostly about the ethical issues related to the use of large scale mathematical models in the new data driven economy. As such, it is valuable both for policy makers and practitioners, since it provides hints of when (self) regulation is due. It is also a relevant reading for consumers who are directly affected by these models, maybe even unknowingly. As the author says, big data is a revolution akin to industrialization, so it is very important to take a look at its potential downsides and not only the possibilities it offers.
I found the book very interesting. The author includes examples from several industries, which helps drive the point, relying more on stories than technicalities. My only criticism is that there wasn't a lot quantification of the effects of the large scale models, just to drive down the point that the problems are generalized. I'm not sure if there has been that much research into the subject though (the author does point out that companies are not too open with the code) , so it probably isn't her fault.
I found the book very interesting. The author includes examples from several industries, which helps drive the point, relying more on stories than technicalities. My only criticism is that there wasn't a lot quantification of the effects of the large scale models, just to drive down the point that the problems are generalized. I'm not sure if there has been that much research into the subject though (the author does point out that companies are not too open with the code) , so it probably isn't her fault.
★ ★ ★ ★ ★
krysty
Great read! I think it is important for anyone who is either A) working as a data scientist/analyst in any capacity or B) interested in how data effects your every day life, from Facebook to Marketing to Banking, to read. Finished it in 3 days. Though it is talking about statistics, it explains its use rather then econometric models. As such, even though with know background can easily digest.
How the Quest for the Ultimate Learning Machine Will Remake Our World :: Iris & Lily: Book One :: Can Make a Positive Difference - How Anyone :: A Modern Fable on Real Success in Business and in Life :: The Art and Science of Prediction
★ ★ ★ ★ ★
paul reed
This book performs a tremendous service. I have a background in science and have completed a couple of statistics courses over the years, but the concept of Big Data is something for which I have a low comfort level. With our increased reliance on computers and the seeming relentless efforts to draw us into more and more data bases, I have come to feel less confident to function in the digital age. This book reinforces those concerns but provides some help in identifying how algorithms integral to both data collection and data interpretation can be manipulated. The concept of using "best practices" has moved from more objectively based studies such as drug efficacy (however, this is hardly immune from data skewing) to education, social work and other areas where the practice advocated can hardly be performed under controlled conditions. But employees are encouraged or required to adhere to best practices because they are based on science. This book gives examples from many areas where the "science" is questionable. Whether applying for a loan, a job or college admissions, the questions asked tend to evoke responses that keep those from lower socioeconomic levels in their place. Another big problem is the influence of the profit motive in determining how algorithms are designed to play out. The ethical implications are huge. As I read the final chapter, I kept being haunted by the thought that this might just be the "silent spring" of the digital age. Many of us may not be aware of the pervasiveness of digital control. And it is becoming increasingly difficult to get a handle on the complex processes manipulating much of what we do. I'm sure many readers with background in computer science will be critical of the lack of depth and specifics. But for those of us who know this is an important problem but need some understandable information, the book is a gift.
★ ★ ★ ★ ☆
kae swu
Excellent book that really uncovers what institutional discrimination is in practice. Good book for classes in Social Work, Human Services, Public Policy and other disciplines concerned with institutional discrimination and oppression.
★ ★ ★ ★ ★
rob silverman
This is a well written book about the data science algorithms that can influence our lives. If all they were responsible for was recommending other books we might be interested in reading, then the topic wouldn't be very important. However, they influence an increasing number of areas in our lives, from insurance pricing, college admissions to hiring decisions, so it is important that the assumptions that underlay these models be explicit and transparent. That's mostly not the case, and in fact, many models take into account data for which it is not legal for a human to take account of. The black box nature of these models is a concern.
I think O'Neil makes a solid argument against the unregulated use of these black box models in society.
O'Neil touches on the issue of the lack of ethics in the tech industry, where smart people are focused on making money and lose sight of the negative impact their work has on much of society.
I think O'Neil makes a solid argument against the unregulated use of these black box models in society.
O'Neil touches on the issue of the lack of ethics in the tech industry, where smart people are focused on making money and lose sight of the negative impact their work has on much of society.
★ ★ ★ ★ ★
jorge ribas
This is a major work!
O'Neil provides the theoretical foundation for many of the positions taken by the Occupy Wall Street movement.
She demonstrates that the Occupy folks have legitimate concerns.
But the philosophical overtones go far beyond protest movements.
She shows how misapplied Big Data analysis can have harmful, if unintended, consequences in many areas of our society.
Don't be put off by the word "math" in the title. The book isn't about math, but rather about common sense.
O'Neil provides the theoretical foundation for many of the positions taken by the Occupy Wall Street movement.
She demonstrates that the Occupy folks have legitimate concerns.
But the philosophical overtones go far beyond protest movements.
She shows how misapplied Big Data analysis can have harmful, if unintended, consequences in many areas of our society.
Don't be put off by the word "math" in the title. The book isn't about math, but rather about common sense.
★ ★ ★ ★ ☆
pam hollern
This is an important book because, in a clear and simple manner, explains the biggest political and legal challenges that the pervasive use of algorithms will increasingly create in our daily lives. As expected, the book presents stats permanently to support its assertions, but I also found useful the anecdotal evidence that Mrs. O'Neil presents. The reason I did not give it 5 stars is that I expected it to go deeper in the proposals or recommendations to address the concern, which are really only analyzed lightly throughout the book.
★ ★ ★ ★ ★
sooriya
No book since Ralph Nader's 1965 classic has exposed as widespread and pernicious a problem as this one does (it is no coincidence that it includes a blurb by him). Cathy O'Neil has now established herself as one the leading authors of our time.
★ ★ ★ ★ ★
katlyn
Very well written book. Very well written analysis of the use of algorithms to determine fundamental decisions and outcomes in our day to day life. Easy read regardless of your level of knowledge of math.
★ ★ ★ ★ ★
nsubuga lule
Interesting book on how big data is being used by so many organizations for many reasons. Highlights the need to instill transparency while maintaining ethical, moral, and legal parameters to ensure fairness and reciprocity amongst organizations and consumers.
★ ☆ ☆ ☆ ☆
melissa
This book tackles an important subject on which the author had a lot of knowledge and expertise, and interesting incisive opinions. Unfortunately it is marred by appalling journalistic lapses, bad enough to taint not just author, but publisher as well. Crown Publishing Group should have done a little fact and reference checking.
The book analyzes a series of mathematical models used for various public and private enterprises. Each chapter is based on a general news account with little additional research. There are a few email exchanges and telephone calls, but only one actual interview, and only one exchange of any kind with someone who builds or defends these models. There are a few other references to blogs or general reference sources, but only on tangential material. Essentially all the information on the models themselves or their effects come from a single popular account from the Washington Post, New York Times, Atlantic, NPR or similar source.
As it happens, I had read all the original sources, and the author cherrypicks only negative information from each. This book should have gone deeper than popular accounts, instead it is more biased and superficial. She does not give specific citations for her information. I went back to try to identify the precise sources. In many cases I can't find it at all, and in many others it is distorted. One particularly offensive practice is to take a quotation from the original piece, re-edit it and change the context, so it means something quite different. A journalist who interviews a source can edit the quote and provide context, generally checking the quote in context with the source, and also making sure to allow anyone who is criticized to have a chance to respond. A later author rearranging words from the same quote and discarding the context, who does not contact the source or give anyone else a chance to respond, is not a journalist. In some important cases, I cannot find anything in the cited article that provides support to the author's assertions in the book.
The most egregious example is the author's account of Mitt Romney's infamous fundraiser video has him calling 47% of Americans takers, rather than saying 47% of Americans pay no income tax. This is in quotation marks, she asserts this is what he said, not what she thinks he meant. However the entire video is available on line, and Romney never uses the word "taker." What he said was bad enough, but the author prefers to make up stuff so that it's more explicitly objectionable. This is not the worst distortion in the book, but it's the most clear-cut because the precise source can be viewed by anyone. In the same section, she claims, "none of the invited donors at the Romney event questioned his assertion that nearly half of voters were hungry for government handouts." Aside from the fact that Romney did not explicitly say voters were hungry for handouts, this statement is clearly beyond the author's knowledge (she gives no source). Did she speak to all the donors? Does she even know who they are? Did she watch the entire video, in which several donors ask questions? This is revealing, because her accusation is clearly not meant to convince anyone, no reasonable person would credit that she knows whether or not any donor questioned Romney's claim. This is a level of dishonesty usually found only in fringe bloggers, religious figures and politicians. There is at least one claim of this sort every few pages in this book.
The first major model discussed in the book is the IMPACT teacher assessment program in Washington, D. C. The account is based on a 2010 series of articles in the Washington Post, describing the first year, plus an email exchange with a teacher who believes she was fired due to cheating by other teachers. Only negative information from the articles is included in the book, and there is no mention of important program changes in the last six years. She claims the mathematical models are "unquestioned" (also without source). This is ludicrous as the program has been under constant attack--political and legal--since inception, and has also been the subject of many academic studies, official investigations and journalistic examinations. I can't offhand think of a mathematical model that has been subjected to more intense questioning. She also claims the models results were not monitored and validated, this is also wildly false, the model has been continuously monitored and validated, and changed significantly as a result. The author claims "you cannot appeal" the IMPACT assessment, in fact there is a strong appeal process, and many teachers have availed themselves of it (the author may believe that the appeals are unfair or ineffective, but she does not say this, nor cite any sources on the subject). The author neglects to mention that IMPACT included intensive in-class observation by human evaluators, feedback and coaching, nor that the human evaluations plus principals' opinions were weighted equally to the test scores. The book gives the impression that the only purpose of IMPACT was to fire teachers, in fact the main purposes were to coach teachers, assign individuals to the best situations and reward the most effective teachers. These are important issues for discussing the program.
Moving from journalistic standards to argument, things get better. The author correctly identifies some problems with both this particular model, and mathematical models in general. For example she notes that the model was opaque. I can't find a direct support for that in her sources, but the Post series did mention that the technical description of the adjustment of student test scores (a key part of the IMPACT model) was not released until more than a year after the program began. This was a serious lapse, and supports the author's charge. However since she stopped reading about the program in 2010, she doesn't mention that the technical report was in fact released, so the model is no longer opaque. Obviously it's very bad to initiate the program before disclosing the details, but this is a complaint about the original introduction of the model, not of the model as it currently functions. It would be reasonable to complain that the technical report is very technical, so although it is available it is opaque to most people, but the author does not begin to discuss this issue. Generally speaking, even the most complex mathematical models are far more transparent than subjective thought processes inside people's heads, but it's also true that lots of people can made sound judgement about how humans decide things, it takes technical expertise to analyze a mathematical model.
The author writes, "attempting to score a teacher's effectiveness by analyzing the test results of only twenty-five or thirty students is statistically unsound, even laughable." This is an essential point, but there is no discussion or source cited. It's not obviously true. For example, suppose that 10% of the variance of student test scores is explained by the teacher and 70% by objective factors that can be measured (last year's scores by the same students, social background, class attendance and so on), and the remaining 20% is not correlated among students within a class including random noise. If that were true then with 25 students, there is a negligible probability that an average or above average teacher would find herself scored in the bottom 2% (the bottom 2% of teachers on the IMPACT rating, which was based 50% on adjusted test scores, were fired). Of course, you might think teachers make less difference, or that the objective factors are less predictive, or that the residual variance is correlated within a class. But these are arguments to make (and all of them have been studied extensively) not things to laugh at.
The author neglects to point out that even a teacher whose students all scored zero on tests needed below average evaluations by humans to be fired; similarly a teacher with the worst possible human evaluations would be fired only if her students got below-average test scores (of course, teachers could also be fired if they got very bad, but not the worst possible, scores in both categories). She calls the firings unfair, but it's not clear what the basis is. As long as the teachers fired were less effective than their replacements (something that seems very likely even with a flawed evaluation program, and has been validated pretty convincingly) then it's fair in the senses that more qualified people got the jobs and that students got a better education. Of course, no program can claim to identify (or even define) the 2% least effective teachers, so you might say it's unfair that, say, a 10th percentile teacher was fired while a less effective teacher was retained. But unless the program were somehow biased, that's just the luck of the draw. A 10th percentile teacher does not have a right to his or her job because not all less effective teachers were fired.
There are important fairness questions, the biggest one is whether the system was rigged to get rid of expensive teachers--senior teachers with high academic qualifications and large increases in pension benefits coming up. Another is how the teachers judged least effective are treated. If you fire them for cause it's not only hurtful to them, it discourages people from becoming teachers in the first place. It would seem preferable to ease them into non-teaching jobs, either in or out of the school system. It's reasonable to ask whether treating professionals in this way--something few other professionals would tolerate--does more damage than any gain from sorting teachers. However, none of these are discussed in the book.
The remaining chapters all follow the same pattern. Unbalanced and inaccurate summary of a popular news account, made up facts and distorted quotes, uneven speculation about mathematical models, and strong but unsupported conclusions.
One basic fairness question is discussed, but in inconsistent ways. One definition of fairness is to treat everyone the same, to but them all in one big category. Another definition is to treat people according to their individual characteristics and actions, not judging them by anyone else. That means putting everyone into a category of one. Both extremes are difficult to implement, so in practice people are generally sorted into larger or smaller categories by FICO score, income, education or other variables. A category that's too broad (like "Asian") puts dissimilar people together and rewards or punishes individuals for actions of unrelated individuals. A category that's too narrow allows invidious discrimination like harsher punishments for crack dealers than cocaine dealers. The question of the proper category system is discussed in nearly every chapter of the book, but is always criticized as being too broad or too narrow without considering the issues with alternatives.
I wish this author would work with a rigorous editor to use her knowledge of data science and modeling to create a useful book. If she would do more research, stick to the facts, cite good up-to-date sources on both sides of questions, speak with the people writing and using these models and force herself to be consistent about the modeling choices; she could write something important. The issues are crucial now, and only increasing in importance. Not many people know as much as the author does about them, and few of those people are as talented at writing. But without balance, professional standards or analytic rigor, the book is worthless mush.
The book analyzes a series of mathematical models used for various public and private enterprises. Each chapter is based on a general news account with little additional research. There are a few email exchanges and telephone calls, but only one actual interview, and only one exchange of any kind with someone who builds or defends these models. There are a few other references to blogs or general reference sources, but only on tangential material. Essentially all the information on the models themselves or their effects come from a single popular account from the Washington Post, New York Times, Atlantic, NPR or similar source.
As it happens, I had read all the original sources, and the author cherrypicks only negative information from each. This book should have gone deeper than popular accounts, instead it is more biased and superficial. She does not give specific citations for her information. I went back to try to identify the precise sources. In many cases I can't find it at all, and in many others it is distorted. One particularly offensive practice is to take a quotation from the original piece, re-edit it and change the context, so it means something quite different. A journalist who interviews a source can edit the quote and provide context, generally checking the quote in context with the source, and also making sure to allow anyone who is criticized to have a chance to respond. A later author rearranging words from the same quote and discarding the context, who does not contact the source or give anyone else a chance to respond, is not a journalist. In some important cases, I cannot find anything in the cited article that provides support to the author's assertions in the book.
The most egregious example is the author's account of Mitt Romney's infamous fundraiser video has him calling 47% of Americans takers, rather than saying 47% of Americans pay no income tax. This is in quotation marks, she asserts this is what he said, not what she thinks he meant. However the entire video is available on line, and Romney never uses the word "taker." What he said was bad enough, but the author prefers to make up stuff so that it's more explicitly objectionable. This is not the worst distortion in the book, but it's the most clear-cut because the precise source can be viewed by anyone. In the same section, she claims, "none of the invited donors at the Romney event questioned his assertion that nearly half of voters were hungry for government handouts." Aside from the fact that Romney did not explicitly say voters were hungry for handouts, this statement is clearly beyond the author's knowledge (she gives no source). Did she speak to all the donors? Does she even know who they are? Did she watch the entire video, in which several donors ask questions? This is revealing, because her accusation is clearly not meant to convince anyone, no reasonable person would credit that she knows whether or not any donor questioned Romney's claim. This is a level of dishonesty usually found only in fringe bloggers, religious figures and politicians. There is at least one claim of this sort every few pages in this book.
The first major model discussed in the book is the IMPACT teacher assessment program in Washington, D. C. The account is based on a 2010 series of articles in the Washington Post, describing the first year, plus an email exchange with a teacher who believes she was fired due to cheating by other teachers. Only negative information from the articles is included in the book, and there is no mention of important program changes in the last six years. She claims the mathematical models are "unquestioned" (also without source). This is ludicrous as the program has been under constant attack--political and legal--since inception, and has also been the subject of many academic studies, official investigations and journalistic examinations. I can't offhand think of a mathematical model that has been subjected to more intense questioning. She also claims the models results were not monitored and validated, this is also wildly false, the model has been continuously monitored and validated, and changed significantly as a result. The author claims "you cannot appeal" the IMPACT assessment, in fact there is a strong appeal process, and many teachers have availed themselves of it (the author may believe that the appeals are unfair or ineffective, but she does not say this, nor cite any sources on the subject). The author neglects to mention that IMPACT included intensive in-class observation by human evaluators, feedback and coaching, nor that the human evaluations plus principals' opinions were weighted equally to the test scores. The book gives the impression that the only purpose of IMPACT was to fire teachers, in fact the main purposes were to coach teachers, assign individuals to the best situations and reward the most effective teachers. These are important issues for discussing the program.
Moving from journalistic standards to argument, things get better. The author correctly identifies some problems with both this particular model, and mathematical models in general. For example she notes that the model was opaque. I can't find a direct support for that in her sources, but the Post series did mention that the technical description of the adjustment of student test scores (a key part of the IMPACT model) was not released until more than a year after the program began. This was a serious lapse, and supports the author's charge. However since she stopped reading about the program in 2010, she doesn't mention that the technical report was in fact released, so the model is no longer opaque. Obviously it's very bad to initiate the program before disclosing the details, but this is a complaint about the original introduction of the model, not of the model as it currently functions. It would be reasonable to complain that the technical report is very technical, so although it is available it is opaque to most people, but the author does not begin to discuss this issue. Generally speaking, even the most complex mathematical models are far more transparent than subjective thought processes inside people's heads, but it's also true that lots of people can made sound judgement about how humans decide things, it takes technical expertise to analyze a mathematical model.
The author writes, "attempting to score a teacher's effectiveness by analyzing the test results of only twenty-five or thirty students is statistically unsound, even laughable." This is an essential point, but there is no discussion or source cited. It's not obviously true. For example, suppose that 10% of the variance of student test scores is explained by the teacher and 70% by objective factors that can be measured (last year's scores by the same students, social background, class attendance and so on), and the remaining 20% is not correlated among students within a class including random noise. If that were true then with 25 students, there is a negligible probability that an average or above average teacher would find herself scored in the bottom 2% (the bottom 2% of teachers on the IMPACT rating, which was based 50% on adjusted test scores, were fired). Of course, you might think teachers make less difference, or that the objective factors are less predictive, or that the residual variance is correlated within a class. But these are arguments to make (and all of them have been studied extensively) not things to laugh at.
The author neglects to point out that even a teacher whose students all scored zero on tests needed below average evaluations by humans to be fired; similarly a teacher with the worst possible human evaluations would be fired only if her students got below-average test scores (of course, teachers could also be fired if they got very bad, but not the worst possible, scores in both categories). She calls the firings unfair, but it's not clear what the basis is. As long as the teachers fired were less effective than their replacements (something that seems very likely even with a flawed evaluation program, and has been validated pretty convincingly) then it's fair in the senses that more qualified people got the jobs and that students got a better education. Of course, no program can claim to identify (or even define) the 2% least effective teachers, so you might say it's unfair that, say, a 10th percentile teacher was fired while a less effective teacher was retained. But unless the program were somehow biased, that's just the luck of the draw. A 10th percentile teacher does not have a right to his or her job because not all less effective teachers were fired.
There are important fairness questions, the biggest one is whether the system was rigged to get rid of expensive teachers--senior teachers with high academic qualifications and large increases in pension benefits coming up. Another is how the teachers judged least effective are treated. If you fire them for cause it's not only hurtful to them, it discourages people from becoming teachers in the first place. It would seem preferable to ease them into non-teaching jobs, either in or out of the school system. It's reasonable to ask whether treating professionals in this way--something few other professionals would tolerate--does more damage than any gain from sorting teachers. However, none of these are discussed in the book.
The remaining chapters all follow the same pattern. Unbalanced and inaccurate summary of a popular news account, made up facts and distorted quotes, uneven speculation about mathematical models, and strong but unsupported conclusions.
One basic fairness question is discussed, but in inconsistent ways. One definition of fairness is to treat everyone the same, to but them all in one big category. Another definition is to treat people according to their individual characteristics and actions, not judging them by anyone else. That means putting everyone into a category of one. Both extremes are difficult to implement, so in practice people are generally sorted into larger or smaller categories by FICO score, income, education or other variables. A category that's too broad (like "Asian") puts dissimilar people together and rewards or punishes individuals for actions of unrelated individuals. A category that's too narrow allows invidious discrimination like harsher punishments for crack dealers than cocaine dealers. The question of the proper category system is discussed in nearly every chapter of the book, but is always criticized as being too broad or too narrow without considering the issues with alternatives.
I wish this author would work with a rigorous editor to use her knowledge of data science and modeling to create a useful book. If she would do more research, stick to the facts, cite good up-to-date sources on both sides of questions, speak with the people writing and using these models and force herself to be consistent about the modeling choices; she could write something important. The issues are crucial now, and only increasing in importance. Not many people know as much as the author does about them, and few of those people are as talented at writing. But without balance, professional standards or analytic rigor, the book is worthless mush.
★ ★ ★ ☆ ☆
dalia
This book is a mix of contrasts making it difficult to rate. Imagine reading a detailed index of every aviation disaster over the past 50 years, what went wrong and why. All factual. By the end of the book you might not ever go near an airplane. Which makes such a book, in the larger picture, misleading, because nowhere does it mention that flying is the safest way to travel.
This is a book about the potential risks of math, algorithms and machine learning. Indeed, more than potential risks - O'Neil gives some actual cases of algorithms gone wrong. But did it really go wrong? The reader cannot know because from policing to employment to every other example, she focuses exclusively on risks without recognizing and balancing against the benefits.
The risks O'Neil mentions are real. And dangerous because errors in data selection and scoring that undermine an algorithm can be subtle enough to escape the notice of mathematicians and engineers applying them. So a book that points out risks of math & algorithms is welcome given all the recent hype. Yet a book that urges people in the industry to use care and vigilance when applying math & algorithms is ironically hypocritical when it doesn't use that same care in presenting its own examples.
This is a book about the potential risks of math, algorithms and machine learning. Indeed, more than potential risks - O'Neil gives some actual cases of algorithms gone wrong. But did it really go wrong? The reader cannot know because from policing to employment to every other example, she focuses exclusively on risks without recognizing and balancing against the benefits.
The risks O'Neil mentions are real. And dangerous because errors in data selection and scoring that undermine an algorithm can be subtle enough to escape the notice of mathematicians and engineers applying them. So a book that points out risks of math & algorithms is welcome given all the recent hype. Yet a book that urges people in the industry to use care and vigilance when applying math & algorithms is ironically hypocritical when it doesn't use that same care in presenting its own examples.
★ ★ ★ ☆ ☆
maddy pertiwi
Thought the concept was great but unfortunately poorly executed by the author. Instead of detailing facts and digging further into the algorithms and data causing systemic discrimination, the author decided to fill the pages of this book with insults to wealthy and ignorant individuals. Made it hard to read after a while.
★ ☆ ☆ ☆ ☆
rachel rust
This book is an extended essay where the author is trying to make a point about how algorithms can be damaging to our communities.
Unfortunately the logic in the book is a dumpster fire. I was astonished given that the author holds a PhD in mathematics... a very logical discipline.
The main thesis of the book is that there are certain conditions for an algorithm in which it can become a 'weapon of math destruction', and tries to show examples of these cases. O'Neil is decidedly anti-big data and anti-modeling in this book.
Here are my main complaints:
1. Her treatment of all of the examples is offensive to the experts who actually do social science in those fields. She clearly has only a surface knowledge of these issues, makes many factual errors, and does not actually know what current social scientists are working on. For example, in the section about policing, O'Neil says that if the Chicago Police Department hired her as their data scientist (!) she could make these biases and issues with the models go away, all while completely oblivious to what current economists, sociologists, and other experts are working on.
2. The claims made by O'Neil in this book are all testable hypotheses, however she makes NO effort to use data to make her argument, and instead relies on scant anecdotes and sweeping generalizations.
3. O'Neil was contradictory as to whether people are the problem or algorithms are the problem. For example, in the section about Starbucks and employee scheduling software she slammed the managers who took control over the algorithm, but then later explained that we don't have enough people actually being involved who adjust the algorithms as necessary... So which is it?
4. She misses the nuance between 'good' and 'bad' aspects of models. For example, when discussing the US News rating system for colleges, she argues that it isn't appropriate to rank schools. Then she goes on to attack for-profit colleges, while failing to acknowledge that the US News rating system can help guide someone who is underprivileged and doesn't have college counselors to tell them that the for-profit colleges are terribly terribly ranked.
5. She needs to look up the word 'arbitrary' in the dictionary. I'll quote the definition here: "based on random choice or personal whim, rather than any reason or system". Many times throughout the book she describes the choices of models in her examples as 'arbitrary'. A model is the exact OPPOSITE of arbitrary. It makes choices based on the defined rules of the program...
6. There is no original content or analysis in this book, beyond her coining of the phrase of 'weapons of math destruction'.
7. I'm confused why people say the book is well written. It isn't. It rambles and often strays away from the thesis.
In short, she does a disservice to the nuance involved with data and algorithms. She identifies some of the important issues near the beginning (e.g. sample size, out-of-sample conclusions, poor objective functions), however, her poor understanding of her examples, and hack-job of an argument is unfortunate and ultimately damning.
Unfortunately the logic in the book is a dumpster fire. I was astonished given that the author holds a PhD in mathematics... a very logical discipline.
The main thesis of the book is that there are certain conditions for an algorithm in which it can become a 'weapon of math destruction', and tries to show examples of these cases. O'Neil is decidedly anti-big data and anti-modeling in this book.
Here are my main complaints:
1. Her treatment of all of the examples is offensive to the experts who actually do social science in those fields. She clearly has only a surface knowledge of these issues, makes many factual errors, and does not actually know what current social scientists are working on. For example, in the section about policing, O'Neil says that if the Chicago Police Department hired her as their data scientist (!) she could make these biases and issues with the models go away, all while completely oblivious to what current economists, sociologists, and other experts are working on.
2. The claims made by O'Neil in this book are all testable hypotheses, however she makes NO effort to use data to make her argument, and instead relies on scant anecdotes and sweeping generalizations.
3. O'Neil was contradictory as to whether people are the problem or algorithms are the problem. For example, in the section about Starbucks and employee scheduling software she slammed the managers who took control over the algorithm, but then later explained that we don't have enough people actually being involved who adjust the algorithms as necessary... So which is it?
4. She misses the nuance between 'good' and 'bad' aspects of models. For example, when discussing the US News rating system for colleges, she argues that it isn't appropriate to rank schools. Then she goes on to attack for-profit colleges, while failing to acknowledge that the US News rating system can help guide someone who is underprivileged and doesn't have college counselors to tell them that the for-profit colleges are terribly terribly ranked.
5. She needs to look up the word 'arbitrary' in the dictionary. I'll quote the definition here: "based on random choice or personal whim, rather than any reason or system". Many times throughout the book she describes the choices of models in her examples as 'arbitrary'. A model is the exact OPPOSITE of arbitrary. It makes choices based on the defined rules of the program...
6. There is no original content or analysis in this book, beyond her coining of the phrase of 'weapons of math destruction'.
7. I'm confused why people say the book is well written. It isn't. It rambles and often strays away from the thesis.
In short, she does a disservice to the nuance involved with data and algorithms. She identifies some of the important issues near the beginning (e.g. sample size, out-of-sample conclusions, poor objective functions), however, her poor understanding of her examples, and hack-job of an argument is unfortunate and ultimately damning.
★ ★ ☆ ☆ ☆
simeon
Interesting statistical info. Her obvious bias'es weaken conclusions and possibly make some of the 'data', suspect so I would classify the book as liberal socio-political propaganda, which is ok I guess. The author has the right to attempt to Influence people's opinions and actions with her own opaque weapon.
★ ★ ★ ☆ ☆
john beeler
Cathy O'Neill makes the same mistakes she attributes to algorithms because she fails to recognize the underlying problems of incentives and greed. She does call these out in some cases, such as teachers cheating on tests to boost their class's scores or Starbucks managers violating corporate policy to boost per employee revenue, but she largely blames the presence of math. It's as if a hammer is responsible for poor handiwork.
A deeper problem is one of innumeracy. The people who entrust these algorithms with decisions are unaware of the failures of these activities. When you measure something, the people affected by that measure will respond to it to benefit themselves. Teachers will cheat on tests, cops will arrest innocents to satisfy quotas, and Standard & Poors will incorrectly label CDOs. These will happen with or without the algorithms.
It's not that these little lies and cheats seem all that bad in isolation. Without the capabilities of big data, no one might ever care. However, big data is able to shine a spotlight on these things. People who blindly use the results of data science are often incompetent in their fields; they don't know how or why things work. Instead of using the results for decision *support*, they use it for decision full stop.
Her line of misthinking is best shown in her "thinking like a data scientist" to make dinner for her family. That's not a big data situation and the tools are inappropriate there. She can poll the population (instead of a limited sample) and get precise and realtime answers directly. Yet, she thinks of this in a big data way. She applies the tools to a situation they aren't suited for. She doesn't need to model anything in the presence of complete and expert knowledge.
These things are tools. It's up to people, with all of their faults, to apply them correctly. But, we know from thousands of years of history, people will never do that well. That there is math involved never had a chance to make that any better despite the cozy feeling of its false impartiality. Cathy O'Neill found out about this too late.
A deeper problem is one of innumeracy. The people who entrust these algorithms with decisions are unaware of the failures of these activities. When you measure something, the people affected by that measure will respond to it to benefit themselves. Teachers will cheat on tests, cops will arrest innocents to satisfy quotas, and Standard & Poors will incorrectly label CDOs. These will happen with or without the algorithms.
It's not that these little lies and cheats seem all that bad in isolation. Without the capabilities of big data, no one might ever care. However, big data is able to shine a spotlight on these things. People who blindly use the results of data science are often incompetent in their fields; they don't know how or why things work. Instead of using the results for decision *support*, they use it for decision full stop.
Her line of misthinking is best shown in her "thinking like a data scientist" to make dinner for her family. That's not a big data situation and the tools are inappropriate there. She can poll the population (instead of a limited sample) and get precise and realtime answers directly. Yet, she thinks of this in a big data way. She applies the tools to a situation they aren't suited for. She doesn't need to model anything in the presence of complete and expert knowledge.
These things are tools. It's up to people, with all of their faults, to apply them correctly. But, we know from thousands of years of history, people will never do that well. That there is math involved never had a chance to make that any better despite the cozy feeling of its false impartiality. Cathy O'Neill found out about this too late.
Please RateHow Big Data Increases Inequality and Threatens Democracy